Leadership
Price And Profit: Homebuilders Walk A Fine Line At A Tricky Time
You may be able to raise prices -- especially as costs surge -- but should you? Algorithms may have one answer. And there may be other choices.
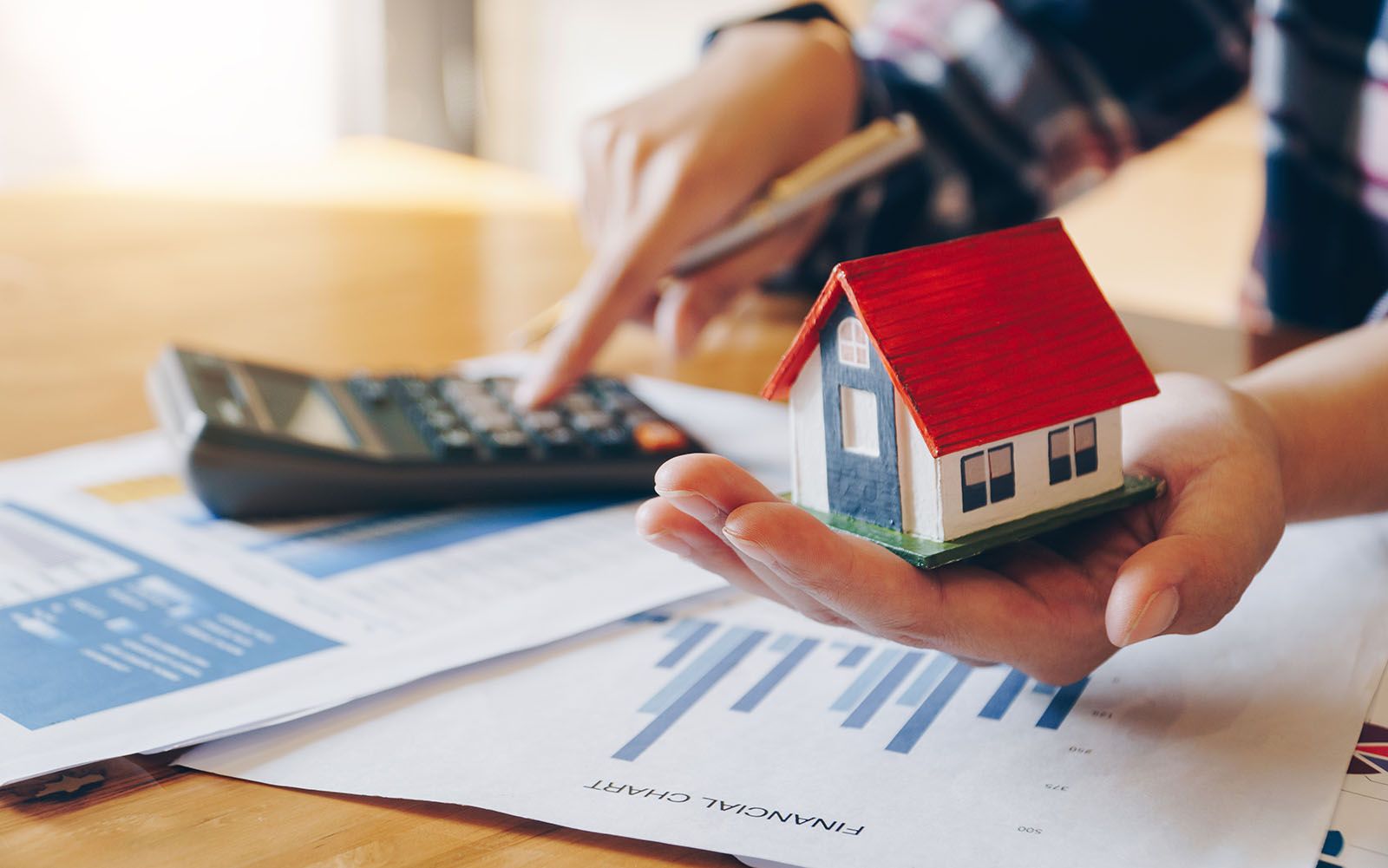
The algorithms were always there, at work.
And since antiquity, their simplicity, elegance, and profound ability to match up important numeric levels and rates of change in the real world to our minds signaled human intellect's highest realms.
Arithmetic algorithms, such as a division algorithm, were used by ancient Babylonian mathematicians c. 2500 BC and Egyptian mathematicians c. 1550 BC.[12]Greek mathematicians later used algorithms in 240 BC in the sieve of Eratosthenes for finding prime numbers,[13] and the Euclidean algorithm for finding the greatest common divisor of two numbers.[14]Arabic mathematicians such as al-Kindi in the 9th century used cryptographic algorithms for code-breaking, based on frequency analysis.
Today, microprocessor- and sensor-powered machines speed up, dig deeper, expand outward, harvest, filter, vet, compare and contrast, winnow out, and sharpen gross absolutes into helpful, defined, and differentiated quantities our minds, our marketing programs, our operational areas, etc. can work with.
In residential real estate, machine learning – paired with human experience, wisdom, and practical realism – elevates what was formerly essentially a trial-and-error business into a far more strategic array of skill-sets and endeavors.
Algorithms triangulate each of residential real estate's pillar economic value measures – demand, utility, scarcity, and transferability – and subject them to heaps and heaps of search, behavioral, preferential, demographic, income, etc. data.
Pace, price, location in all of their abundant themes and variations, external factors such as interest rate basis points, economic indicators, confidence benchmarks, macro data galore now all run through sausage-making multivariate regressions built with complex algorithms precisely to sense and detect deltas of change, trends, rates-of-rates, and the like, and that would be complex enough for any single firm or business sector.
But that's only the half of it.
Consumers – households and residents and would-be renters and owners with preferences, attitudes, behaviors, means, future earnings potential, lifestage priorities, values, ambitions, cultural mores, community attachments, personal and family networks – are a whole other ball of wax for our machine-learning enabled algorithms to get their enormous brains around.
Here's some insight I believe might impact how you approach one of the trickier dimensions of your businesses right now, especially as input cost inflation presses hard on unit gross and net margins.
This piece in the Harvard Business Review's [pay-gated] September-October 2021 issue, comes from two marketing professors, Marco Bertini and Oded Koenigsberg, could not be more timely for companies that face Hobson's Choice challenges to put customer trust at least on a par with near-term gains in profitability.
Pricing algorithms are intended to help firms determine optimal prices on a near real-time basis. They use artificial intelligence and machine learning to weigh variables such as supply and demand, competitor pricing, and delivery time. Unfortunately, algorithms occasionally go rogue and come up with figures no one would ever pay—from $14,000 for a cabinet listed on Wayfair to almost $24 million for a textbook offered on Amazon. But such snafus are just one of the risks when companies entrust decision-making to computers.
The constant changes in price points send strong signals to customers that need to be properly managed. Yet many organizations fail to appreciate this. They know that prices affect decisions about when and what to purchase, but they overlook the fact that continual ups and downs may trigger unfavorable perceptions of their offerings and, importantly, the company itself.
Brands thus need to consider more than simple math when employing algorithmic systems. These systems can create an uncomfortable tension between earning customer loyalty and earning money.
The conclusions Bertini, who hails from at Esade–Universitat Ramon Llull, in Barcelona, and London Business School professor Koenigsberg reach track directly to common sense and golden rule principles and practices. Many insights – like these – have that "I wish I thought of that" characteristic.
The take-away is this. The profound and growing opportunity in machine learning comes not just in velocity, expanse, depth, precision, and usefulness. It's also simply this notion, the one that stretches back to antiquity, that learning itself is constant and new and uncharted. Wisdom, experience, handed-down knowledge, and even cleverness are not enough right now to fathom what will build and reinforce our customers' our team member associates' and our partners' trust and confidence that we have their backs.
Join the conversation
MORE IN Leadership
Offering Builders Certainty In An Uncertain Home Insurance Climate
Explore how embedded insurance solutions empower builders to address homebuyers' affordability and coverage concerns amid rising climate risks.
Builders Foresee ‘Tough Slog’ In Next Admin's Housing Promises
With housing shortages in every U.S. county, builders brace for policy shifts and market dynamics that could shape the future of affordability, access, and demand. Here's a business leader's playbook.
Climate Risk Is Reshaping The New Home Buyers Landscape
Helping Builders and Buyers Navigate High-Exposure Markets