Technology
AI's Fast Learning Curve On Pace To Impact Value Cycle
There's hardly a clearer example of this vector's to-date trapped opportunity – humans, dirt, and bots – than in the single-family-for-rent and build-to-rent real estate platform.
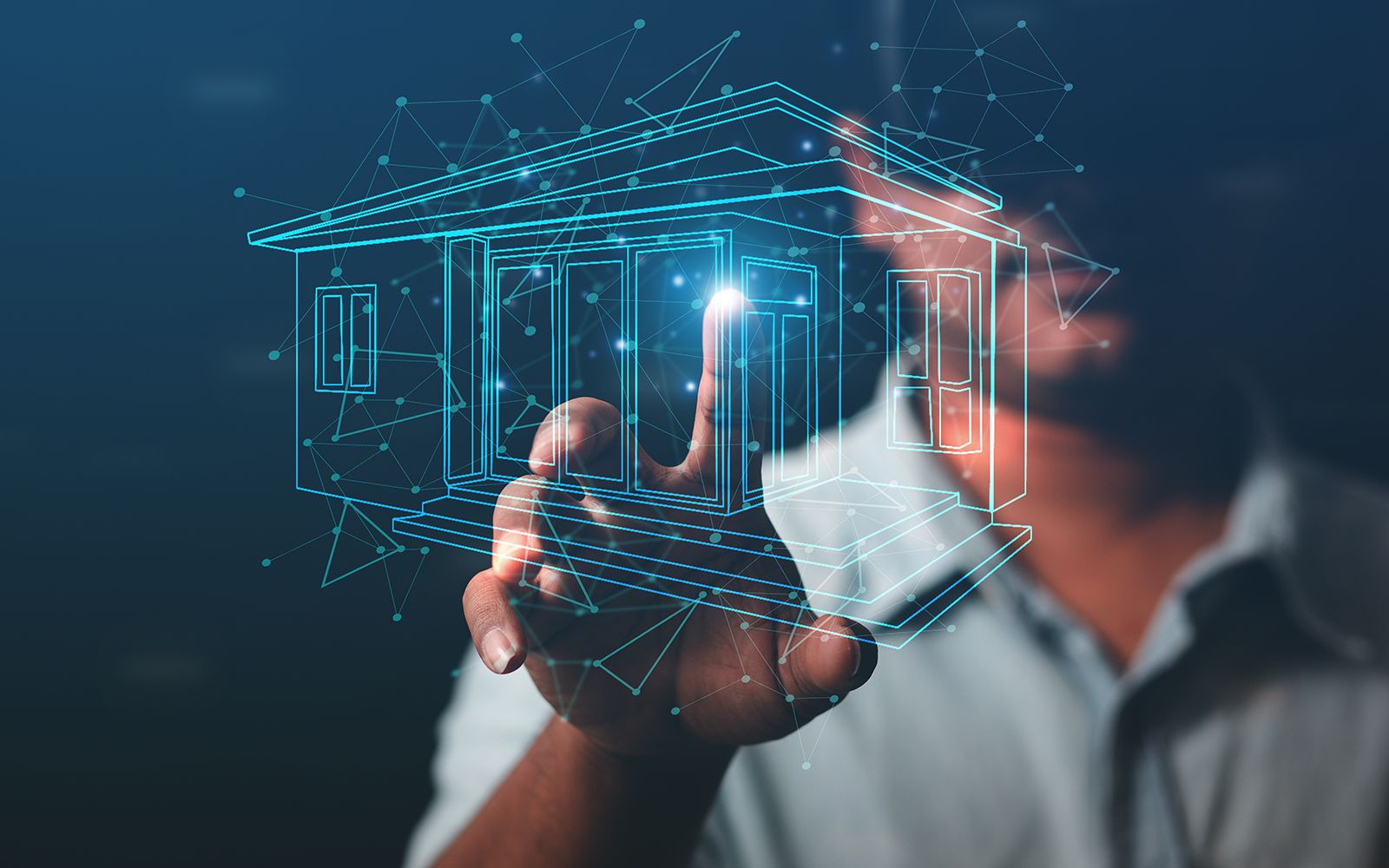
So, Google's Bard this week experienced its first major tail-between-its-legs moment on a grand stage of global scrutiny. We confess, we think the better of Bard – and, for that matter, the entire newly-minted 'Gen B' chatbot cohort – for it. Here's why.
So what if machine learning's got a learning curve just like the rest of us. Bard stepped up into the arena and fearlessly gave the wrong answer.
When was the last time you saw a human make a $100 billion mistake? ... Bard’s confident wrongness isn’t uncommon in generative AI—ChatGPT’s ability to be boldly inaccurate is well documented. The systems work by analyzing patterns in a huge mass of text and making predictions about what words come next (rather than analyzing and synthesizing ideas, like our brains do). This has led one Princeton professor to refer to them as 'bullsh*t generators.'” – Max Knoblauch, Morning Brew
C'mon, who doesn't love "confident wrongness?" And who wouldn't give that smarter-than-its-years whippersnapper of a chatbot a good, encouraging kick in the chat-butt with words to the effect of ..."there'll be other world-wide moments of truth, so put this one behind you and move on. Prepare better next time! You'll go far!"?
After all, if to err is human, what wouldn't the same hold true of AI? Isn't the construct of intelligence at least somewhat human, artificial or not?
Anyway, the point is even as the practical, democratized potential of AI has seized our collective imaginations with the supernova of the OpenAI ChatGPT's marriage with Microsoft platforms igniting the chatbot space race, "confident wrongness" is a thing. Looking back at the 2020s from another time, Bard's $100 billion brain-freeze may be remembered as a quaint blip in the rankings of chatbots biggest blunders.
Who knows?
What we do know is that while a tech stack of language, knowledge, data, semiconductors, etc. have taken operations lightyears ahead of where they were a decade ago, stumbling blocks – i.e. "analyzing patterns in a huge mass of text and making predictions about what words come next (rather than analyzing and synthesizing ideas, like our brains do" – remain.
For now anyway, particularly where technology, data and people, and technology, data, and dirt all meet, bots need us, and we can benefit from them.
There's hardly a clearer example of this vector's to-date trapped opportunity – humans, dirt, and bots – than in the single-family-for-rent and build-to-rent real estate platform.
- investors embraced it as an asset class during the great financial crash, housing meltdown, and foreclosure crisis
- households embraced it as a housing preference channel – for financial reasons and by-choice – in the decade plus since
- residential community development, builders, and pure-play BTR operators embraced it as a sustainable growth business mostly in the past 8 or 9 years.
Data and technology played critical roles in that trajectory. In a lengthy interview with Bloomberg Markets staffer Sonali Basak, Pretium Partners founder and ceo Don Mullen sets the context for the single-family-rental sustainable business model:
In the single-family rental business, in particular right now, people—ourselves and Invitation Homes, American Homes 4 Rent, Tricon [Residential] and others—have proven the ability to scale the purchase of the assets and operate them at attractive margins. The risk of investment is lower, because you don’t have uncertainty about operational effectiveness."
Mullen elaborates, likening the opportunity and its sustainability as a model, to the credit derivatives business he strived and thrived in in his life before Pretium.
Everybody in the real estate business thinks every piece of real estate is unique, every house is one of a kind. Snow is a perfect metaphor for this. Every snowflake is truly unique, but when you put a lot of it together, it’s just a white pile. We said, “What do we want to buy?” We want to buy a 2,000-square-foot house built in the 21st century, preferably in a homeowners association, with a two-car garage, a front and backyard, not on the corner. Now you just unleash the computer to analyze every house for sale in 22 markets, every 15 minutes. And it’s like equity trading with computers, right? I had, like, the Citadel of houses.
We have, as a country, a lot of alternative data, which helps. I’ll give you one example. Every single house we buy, we also score that area by the average FICO score of everybody who lives in that community, the crime rate, the average commute time for a person living in that house to a place of work, average school scores. There’s an optimization curve—people are willing to pay up only to a certain point for a school district. People in America don’t want great school districts, they want average-plus. We spend a lot of time identifying long-term demographic trends: What do we expect the growth rate of the population to be? What’s the average income of the people moving to that city? Because then you can predict both housing demand and at what price point."
Mullen, thanks to the data, the capital, and the disciplines and rigors of his team's investments has staying power to weather periods of turbulence and uncertainty. The bots can get things wrong. He and his team are course correcting, de-risking, and patient and the plan is to be there as structural demand – people moving, new households forming, and families forming – resumes.
Just an example of where machine learning can do a lot of the work it once took time and people to do. And then there are people who keep that machine learning's learning curve going where it has a chance to get the right answer after all.
MORE IN Technology
Trailblazers 2025: LenX Teams Up With Base On Home Energy Breakthrough
Lennar's partnership with Base Power delivers homebuyers backup energy, affordability, and resilience — an industry-shifting innovation powering up in deregulated Texas markets.
Building Smarter: Adapting To 2025's Top Housing Challenges
How technology, data, and efficiency are transforming homebuilding amid affordability pressures, demographic shifts, and evolving market demands.
Tech And Data's Role In Shaping A New Normal For Homebuilding
Essential data-driven tools and long-term partnerships help builders navigate today’s volatile housing market. Discover how technology empowers builders to compete in a rapidly changing industry.